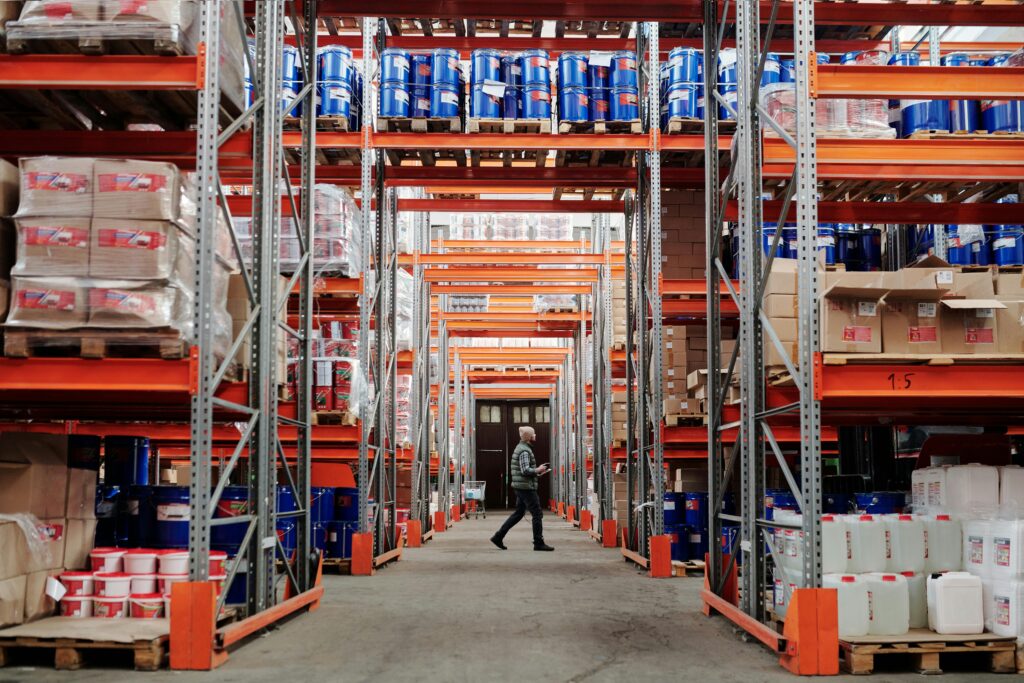
The adoption of artificial intelligence (AI) is already transforming supply chain operations, allowing products to be kept in stock on store shelves, optimizing delivery routes and doing even grander things like identifying risk in a global supply chain to avoid disruptions.
The global market for AI in the supply chain — incorporating such technologies as machine learning, predictive analytics, and automation — is estimated to grow to $40.53 billion by 2030, up from $9.15 billion in 2024, according to management consulting firm MarketsandMarkets. AI and its related technologies are expected to bring greater end-to-end efficiencies to supply chains.
For example, machine learning technology (not all AI-powered applications use machine learning) leverages large datasets to improve efficiency and decision-making across the supply chain, enabling systems to learn and adapt without being explicitly instructed.
Here’s a look at five ways that AI technologies are helping to transform supply chains:
Inventory management
AI facilitates seamless inventory management across channels, from stores to online orders and in-store pickup. Many retailers are giving consumers the capability to see stock availability and quantity of an item at the store level. AI used for inventory management helps retailers optimize stock levels, reduce waste and improve customer satisfaction. Combined with machine learning, retailers can analyze vast datasets quickly and easily, allowing them to initiate stock replenishment based on demand. Larger, more sophisticated retailers use machine learning to automatically replenish stock based on sales patterns, supplier performance and shelf life.
In the warehouse and distribution center, AI-driven warehouse management systems can track inventory levels in real time, optimize picking routes and storage space, bringing improved accuracy and throughput rates, among other benefits. Combined with other AI-powered automation technologies such as automated storage and retrieval systems (AS/RS) and autonomous robots, discrepancies are reduced and customer satisfaction improves.
Predictive demand forecasting
One natural outgrowth of AI-enabled inventory management is more accurate demand forecasting. AI can render more detailed forecasts at the product level, enabling retailers to optimize inventory management, thereby reducing stockouts and overstocks. Machine learning models identify patterns and relationships between variables, enabling more accurate demand predictions.
From the supplier perspective, on-time in-full (OTIF) rates are one of the most critical KPIs: delivering the right product in the right quantity at the right time to the retailer. Mismanagement of the OTIF performance metric can cost a large supplier millions of dollars annually.
Large consumer product companies are among those suppliers using AI and machine learning to analyze historical sales data, market trends and other factors. AI algorithms can crunch vast amounts of historical sales data, seasonal trends and external factors like weather, holidays and economic indicators to provide more accurate demand predictions. Continuous learning and improvement allows adaptation to new data and refinement over time.
Route optimization
Some 25 years ago, real-time traffic information via GPS tools began to emerge and now the technology is ubiquitous on phones and embedded in many software tools serving the logistics industry. AI-driven route optimization offers next-level benefits by truly optimizing routes to analyze real-time data like traffic conditions, weather forecasts and delivery locations to calculate the most efficient delivery paths.
AI-powered route optimization means routes can be dynamically adjusted on the fly to avoid delays, minimizing travel time and fuel costs, all while considering factors like vehicle capacity, driver availability and delivery time windows. It’s a win-win for operators and customers alike.
RFPs and bids
For shippers and those in the supply chain requiring logistics services, the creation of RFPs has streamlined over the years with online sourcing tools and procurement software, though largely remains a manual process.
According to Accenture, challenges of RFPs include incorporating data from multiple sources in multiple formats, requiring cleaning of data to remove anomalies. AI can bring machine learning algorithms to better analyze historical data such as past bid outcomes, pricing trends and supplier performance, giving creators valuable insights while helping to identify anomalies in data for evaluation. All of this produces more targeted bids with forecasting accuracy. AI also allows shippers to better evaluate bid responses, rendering insights for decision-making.
On the flip side, logistics companies can respond to an RFP with more confidence when validating their assumptions by using AI-powered tools to analyze the RFP document, identifying key requirements, evaluation criteria and deadlines. Responders can also strengthen their bids using data-driven insights and analytics on the RFP requirements so they can quickly identify key differentiators and develop their own targeted solutions, and then quantify the benefits with ROI which can help set them apart from their competitors. Lastly, logistics companies can better manage risk: AI tools can support compliance with the RFP requirements, thereby reducing errors, omissions or non-compliance.
Workforce automation
The U.S. logistics industry continues to struggle with labor shortages to fill essential roles such as warehouse workers and truck drivers. In the warehouse, the adoption of AI-powered automation technologies such as automated forklifts and other advanced material handling systems are helping ease the burden.
Greater use of AI is already enhancing human productivity with AI tools put in the hands of workers on devices. The National Bureau of Economic Research Report found that about 28 percent of all U.S. workers are using generative AI on the job.
For warehouse workers, AI-enabled applications on wearables, handheld and portable devices are increasingly used for real-time inventory checking, order picking guidance, optimized picking routes, barcode scanning with error detection, predictive maintenance alerts, training and support and data collection for performance analysis. These devices are aimed at improving efficiency, accuracy and worker safety within the warehouse.